A sweet lyrical revolution is playing out at IIITH labs. The Cognitive Science laboratory worked on a Natural Language Processing-based Transformer model to identify emotions from the lyrics of a song, a hitherto underexplored area of study. We met with the team to learn more.
Imagine you are navigating through dense traffic, while an online music streaming platform plays in the background. You are skipping through your playlist, just to get to that one song that will match your mood. Today, online music streaming platforms are rewriting the dynamics of the music industry. Paid subscriptions to a plethora of online music streaming platforms has risen dramatically, especially in the pandemic era. This spotlights the relevance and applicability of robust Music Information Retrieval (MIR) systems.
Music transcends global borders and cultural idiom, to express moods and feelings, without language. Individuals seek a varied range of emotional experiences and gratification via music. The obvious and subliminal effects of music on physical and mental homeostasis has, for long, been a topic of study. Music Emotion Recognition (MER) is a finely nuanced subfield of MIR which aims to identify and classify music emotions from musical features including genre, artist, acoustic features, social tags, amongst others. Music streaming services are now able to recommend music based on mood, time of day and purpose (e.g., gaming, cooking and dining). However, lyrics, which are a key element in music, have largely been neglected and underutilized in the field of Music Information Retrieval.
At the recently concluded 43rd European Conference on Information Retrieval at Lucca, IIITH researcher Yudhik Agrawal presented his findings on the subject. What set it apart was that it was the first study to use the emerging Transformer-based approach towards Music emotion recognition from lyrics.
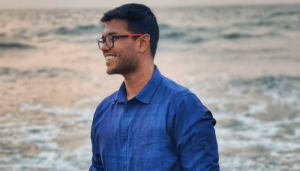
Lyrics and MER
Would it feel the same if you listened to your favorite song, but without the lyrics? Probably not. “The role of lyrics in MER remains under-appreciated, in spite of several studies reporting superior performance of music emotion classifiers, extracted from lyrics” observed Yudhik Agrawal.
“This study actually resulted as a by-product of a specific task that the students were working on for another I-Hub data project”, remarked Prof. Vinoo Alluri, the Cognitive Science Advisor on the team. Given the premise that music is a coping mechanism, they were trying to qualify and quantify the lyrics that resonate with people who are prone to anxiety and depression. Thus began the exercise to categorize lyrics.
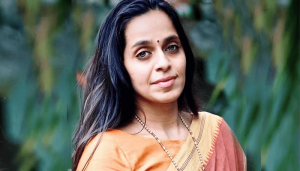
Music emotion recognition has typically relied on acoustic features, social tags and other metadata including artist information, instrument and genre data to identify and classify music emotions. Lyrics, despite being a vital factor in eliciting emotions, is predominantly an untapped domain. In earlier music emotion classification systems, the words in lyrics were traditionally linked with lexicons that possessed a limited vocabulary, and emotional values were assigned to them without using avana 50 mg any contextual information. Even with recent MER techniques, which involve pre-trained word embeddings and sequential DL models (RNNs), there is an unavoidable context-relevant information loss while establishing longer dependencies.
XLNet Transformer rejigs the system
Technological advancements in the field of Natural language processing (NLP) have made available a host of techniques that could be used in the field of Music Information Retrieval to analyze lyrics and extract relevant features such as topics, sentiments, and emotions. Along with Ramaguru Guru Ravi Shanker and Prof. Vinoo Alluri, researcher Yudhik Agrawal has been experimenting with the transformer-based approach using XLNet as the base architecture. To the uninitiated, XLNet is a relatively new deep-learning based technique for language modelling in the booming field of NLP.
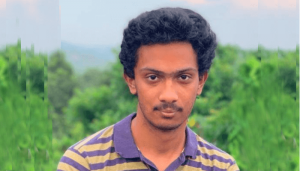
The first step to classifying lyrics based on emotions was to identify the framework for categorizing emotions after which Deep-Learning approaches could be employed. Emotions can be either projected on a two dimensional “Valence-Arousal” (V-A) emotional space wherein Valence represents the pleasantness and Arousal represents the energy dimension. In addition, they can also be categorized into discrete emotion classes representing “joy”, “anger”, “sadness”, and “tenderness”. For instance, positive Arousal and positive Valence represents the quadrant associated with Happiness related emotions, while negative Arousal and negative Valence represents the quadrant associated with Sadness”, elaborated Yudhik. An NLP-based transformer model was then designed to predict Valence and Arousal in addition to the four emotion classes, given the lyrics of a song as input.
Nuts and bolts of data extraction
“We stumbled upon a couple of annotated datasets that contains 180 songs, distributed uniformly among the 4 emotion quadrants”, observed Yudhik. The Valence – Arousal values assigned for each song was solely based on the lyrics displayed, without the audio. The V-A for each song were computed as the average of their subjective ratings. The other dataset, MoodyLyrics, is a much larger dataset which contains 2595 songs, uniformly distributed across the same emotion quadrants of V-A space. The values for valence and arousal were assigned, using several lexicons like ANEW.
Given these datasets, the next challenge was to mine lyrics since the datasets do not provide lyrics due to copyright issues. Moreover, the provided URLs become obsolete over time. Hence, lyrics extraction had to be done with the help of Artist and Track details. Existing APIs, including Genius require the exact artist/track name for extracting the lyrics, which is frequently misspelt in the datasets. “To overcome this hurdle, we chose to use the Genius website and an added web-crawler, a simple tweak, which helped in getting the correct URL using Google search”, observed Yudhik.
Tapping into the lyrical soul of emotion recognition
Results of the Study indicate that the state of the art Transformer model outperformed existing methods for multiple datasets. “This study has important implications in improving applications involved in playlist generation of music based on emotions. For the first time, the transformer approach is being used for lyrics and is giving notable results”, reported Yudhik. “In the field of Psychology of Music, this research will additionally help us in understanding the relationship between individual differences like cognitive styles, empathic and personality traits and preferences for certain kinds of emotionally-laden lyrics,” said Prof. Vinoo Alluri.
In fact, in the upcoming 16th International Conference on Music Perception and Cognition, Yudhik will be presenting an extension of this work that uses this model and relates it to individual traits (personality) and their preference for certain kinds of (emotions via) lyrics as it occurs “in the wild” (i.e., on online music streaming platforms). In addition, two other students, Rajat Agarwal and Ravinder Singh, working with Prof. Alluri, will also present their work that incorporates Yudhik’s model into a new deep learning based classification paradigm called capsule networks.
“Looking ahead, our objective would be to get a granular understanding of music-seeking behavior in depression or anxiety-prone individuals by understanding their lyrical preferences and extracting more specific nuanced emotions. For instance, when you say sadness or grief, the emotions can range from Sweet-Sorrow, all the way to Nostalgic-Longing” said Prof. Vinoo Alluri. Now that we have an English-language based MER system, it would be nice to collaborate with our Language Technologies Research Centre to expand the scope and scale of the research, to incorporate the rich musical legacy that is present in our regional Indian languages” mused Prof. Alluri. Now, that’s music to our ears!
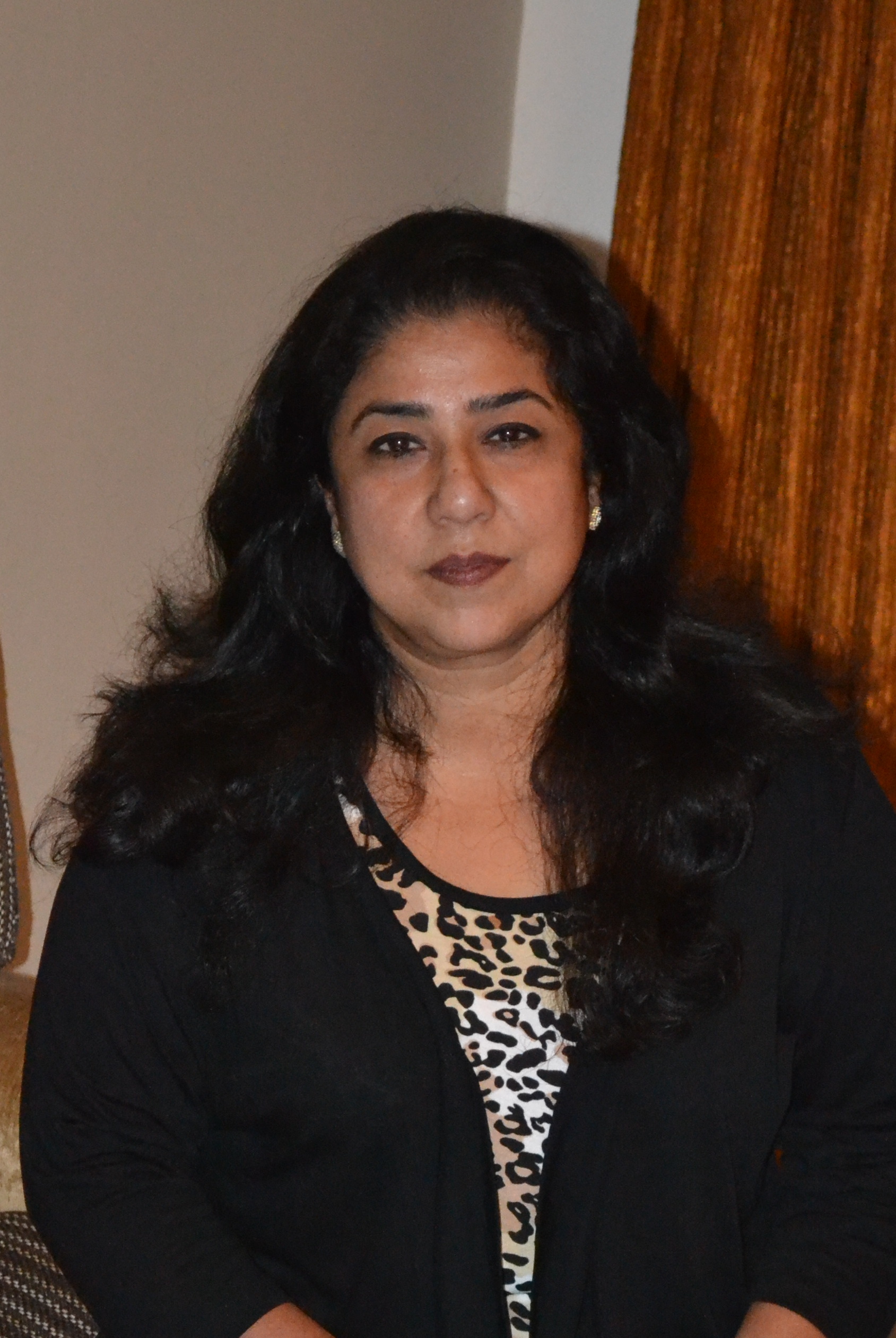
Next post