At a time when the country is grappling with a severe Covid resource crunch, IIITH researchers show how ML models can help in prioritizing healthcare based on risk and mortality prediction.
The more we learn about the Coronavirus, the less we know. With the virus affecting different people differently – from showing no symptoms at all or mild and moderate requiring no hospitalization, to the sudden onset of severity such as breathlessness and confusion, it’s become imperative to know early on in the diagnosis about the progression of the disease. As part of a joint project funded by Intel Corp, under its Pandemic Response Technology Initiative along with CSIR-IGIB (Council of Scientific and Industrial Research – Institute of Genomics and Integrative Biology), researchers from IIITH have used machine learning models to categorise risk and predict mortality in Indian patients.
Existing Studies
Machine learning-based algorithms that analyse COVID-19 patients’ data and provide disease prognosis are not entirely novel. Different groups of researchers have created mortality prediction models based on variables such as age and varying biomarkers derived from blood samples. For instance, one of the earliest studies was conducted by Xie et al which looked at oxygen saturation, age and other biomarkers to generate a prediction model. Shortly thereafter, Yan et al used the popular and more efficient XGBoost algorithm on three blood sample features. The solution they derived was 90% accurate in predicting mortality across all days of being diagnosed Covid positive. “It’s easier to have a more accurate prediction the closer you are to the day of the outcome,” says Akshaya Karthikeyan, IIITH researcher from the Centre for Computational Natural Sciences and Bioinformatics (CCNSB), explaining that ‘outcome’ refers either to getting discharged or death. She is the lead author of the paper titled “Machine Learning Based Clinical Decision Support System For Early Covid-19 Mortality Prediction” that has attempted to provide a mortality prediction as early as 16 days before the outcome. Using the same dataset of Covid positive patients from Wuhan, Akshaya and her team identified 5 biomarkers that can be used to predict mortality with 96% accuracy. According to these researchers, an early prediction can help accelerate the decision-making process of healthcare professionals for appropriate treatments.
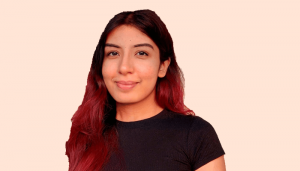
Indian Patients
While all these algorithms provide much-needed insights, the biggest drawback is that they have been trained on patient data obtained either from China or the US of A. With individual disease response varying not only due to ever-mutating strains of the virus, but also population characteristics and different hospital practices, such studies cannot be universally applicable. In a bid to fix this flaw, the CCNSB researchers conducted an India-specific study – this time on 544 Covid positive patients from the MAX group of hospitals in New Delhi. Putting out a disclaimer that the study (currently under peer review) was conducted during the first wave of the pandemic in India, Shanmukh Alle, lead researcher of the effort says that apart from predictive modelling for the Indian populace, they also wanted to investigate plausible factors behind the low mortality rate in Indians as compared to that of the Chinese.
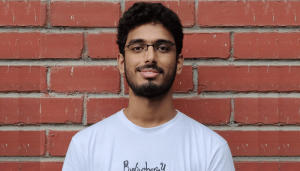
What They Did
First, the researchers tested out the neural network created by Akshaya on the Indian dataset. Unlike the high (96%) accuracy of mortality prediction demonstrated in the early stages of COVID-19 diagnosis, they found that Indian mortality could be predicted with an accuracy of only 58%. One of the biggest puzzles was that Indian patients who were at high-risk and expected to die based on the Wuhan dataset actually survived. Evidence was found linking mortality with the usage of steroids. This is in line with the early treatment protocols by the Indian government mandating the use of steroids and immunosuppressant drugs. Predicting patients’ risk, that is, if they are at high-risk or low-risk based on respiratory support needed (if at all) is important in order to effectively allocate scarce resources. For this, biomarkers such as blood parameters, oxygen saturation levels and diabetes comorbid conditions were identified. For mortality prediction however, only blood parameters were considered. Two different machine learning methods were used for risk stratification and for mortality prediction, respectively, both of which have yielded very good results.
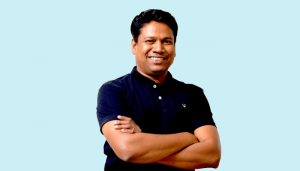
Next Steps: Genome Sequencing
With the study identifying high-risk patients and thereby focusing on relevant treatment, it is one way towards helping countries that are tottering under an inadequate and heavily overburdened healthcare infrastructure. Lauding the joint efforts of a large number of researchers from IIIT Hyderabad, Intel, Max Hospitals and CSIR-IGIB who came together to undertake this on a large scale in a timely fashion, Prof. Deva Priyakumar makes a special mention of Dual Degree students Shanmukh Alle, Akshaya Karthikeyan and Akshit Garg: “They rose to the occasion to push this effort towards developing the clinical decision support systems for risk stratification and mortality prediction of COVID-19 positive Indian patients.”
Akshit Garg
With the current surge in cases in India being linked to a particular variant of the COVID-19 virus, its mutations are being tracked closely. “Currently, Shanmukh and another MS student Ruchi are analyzing the viral genome collected from the Indian patients to find mutations that may be associated with severity of the COVID-19 disease”, says Prof. Deva. According to Dr. Vinod P.K who is leading this initiative at the CCNSB, “Mutations in SARS-CoV-2 are mostly linked to the increased infectivity of the virus. Linking it to the severity of disease requires further understanding of how the mutant virus interacts with the host factors and comorbid conditions. In addition to sequencing of viral genome, there is also a need to sequence the DNA of SARS-CoV-2 infected patients with different outcomes.”
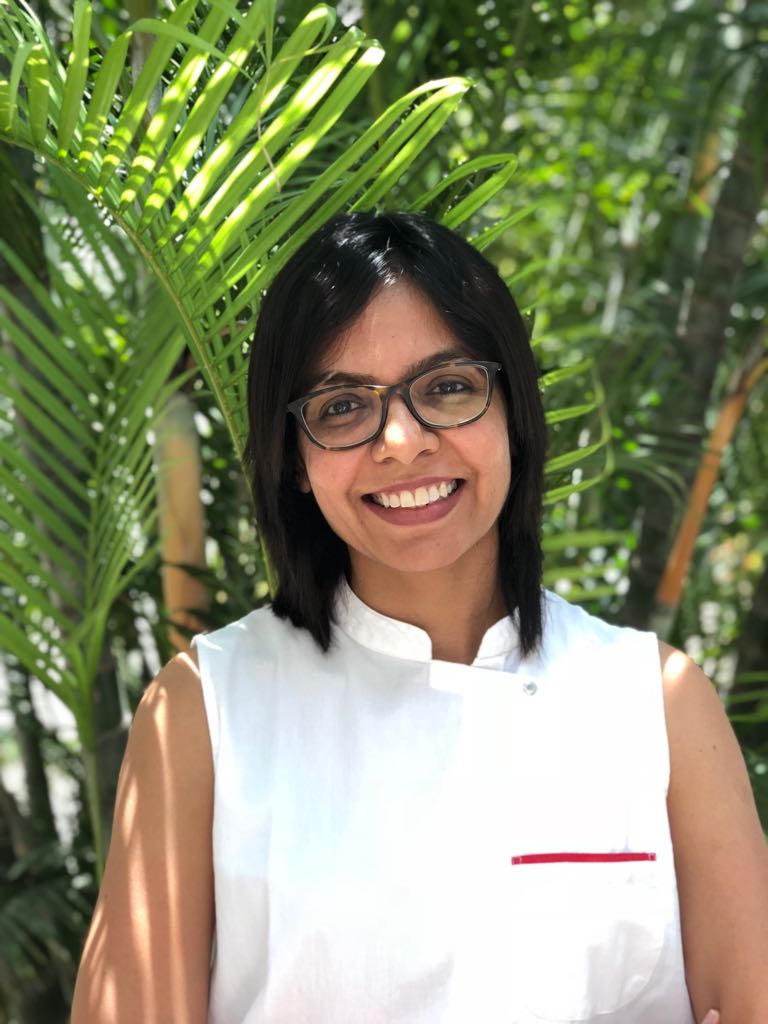
Sarita Chebbi is a compulsive early riser. Devourer of all news. Kettlebell enthusiast. Nit-picker of the written word especially when it’s not her own.
Next post