From assisting experts in healthcare diagnosis based on images alone, AI-assisted radiology is evolving to include multiple evidence-based reports, observes Prof Jayanthi Sivaswamy.
Images have been a key part of diagnosis in healthcare for long. From the X-ray that is routinely used to screen the chest (for a variety of diseases) and the breast (for cancer), to ultrasonography for assessing the health of a foetus or the abdomen, heart, etc, to MRI/CT for detecting strokes and haemorrhages, a wide range of imaging options are employed.
Ease Of Digital Imaging and Availability of Expert Diagnosis: A Mismatch
The advent and widespread use of digital computers spurred even film-based imaging such as in X-ray to go digital, facilitating not only easy storage but also processing by computers. Visual exams of the retina by ophthalmologists and of biological specimens on slides by pathologists also have digital footprints now, due to advances in digital cameras and their easy availability. This enabled telemedicine as digital images taken at one location can be easily transmitted to an expert far away for either real time or off-line consultation. However, there is a bottleneck. The increasing ability to image is however highly mis-matched with the availability of medical expertise needed to read and interpret the images. Systems that automatically process medical images are expected to offer a solution to address this gap as well as increase efficiency in clinical workflow by providing assistance to experts. Machine learning from images is a key methodology enabling this automated processing.
Evolution Of AI in Medical Imaging
The goal of AI systems designed to process images has become increasingly ambitious. Initially it was to primarily assist experts in diagnosis. The earliest approval given by the Federal Drug Authority or FDA for such an automated solution in breast cancer screening was in 1998 as a second reader. I.e., a human expert had to first read the mammogram and record their decision on the presence of cancer; only after this were they allowed to access the view of the AI system and possibly modify their original decision. With increasing maturity of technology and proven performance, standalone systems are also part of the goal especially in screening for diseases.
AI-assisted medical device is a broad category where ML algorithms are part of a medical device. An example is a wearable sensor paired with a special software running on a mobile phone for monitoring epilepsy. There has been a rising number of regulatory approvals in this category. In the last 5 years, the Federal Drug Authority or FDA in USA has also issued approvals for another special category, namely, software as a medical device or SaMD. This has been predominantly in the image-based area of Radiology, with 531 approvals given in 2023 which is an impressive 77% of all approved medical devices.
Many of the FDA-approved-SaMDs are targeted at aiding clinical workflow and emergency medicine. Examples of the first kind are those proposed for detecting stroke / intracranial bleeding, cancers of the lung, liver and breast, and cardiovascular analysis; examples of the second are devices for detecting pneumothorax or collapsed lung, rapid triaging of time-sensitive cases and wrist fracture. Devices have also been approved for use in improving general workflow in areas like neurology and ophthalmology. The latter has the distinction of being the first area in which a fully standalone device was approved for detecting diabetic retinopathy – a sight-threatening condition if untreated.
Deep Learning In Image Computing
The recent uptick in SaMD for Radiology is due to the developments in machine learning in general and computer vision in particular. Machine learning underwent a paradigm shift to deep learning (DL) which focuses on intelligent processing of general images. The hallmark of DL is that learning is done by neural networks which are computational schemes inspired by networks found in our brain; the learning is done directly from data unlike features in traditional machine learning where the features were generally handcrafted based on the task at hand and extracted from the data. The paradigm shift led to huge success in Computer vision, paving way for numerous everyday applications from biometrics-based secure access to smartphones and airport terminals to driverless cars. These advances also spurred data driven methodologies to be adopted in medical image computing for automating specific tasks resulting in SaMD.
The initial success of AI-assisted Radiology led to so much exuberance that some experts even predicted a doomsday for radiologists. However, the last five years have shown that this is only hyperbole. This is due to multiple reasons, a fundamental issue being the data driven nature of DL-based systems and the complexity of the practice of medicine.
What is the model learning?
A research study done in our group at IIITH looked at this question for Pneumonia which is a disease affecting the lungs. It can be caused by fungal, bacterial and viral infections each requiring very different type of treatments. Typically, chest X-rays are used along with other lab tests for the differentiation. During COVID 19, numerous papers were published to detect COVID-pneumonia from X-rays; they reported very high detection accuracy. When we queried the algorithm on which part of the chest X-ray image was the basis for a positive decision, regions outside the lungs were shown in many cases! This does not instil confidence to adopt such a model in a clinical workflow since it appears to perform a task well but by learning something from the image data that is not anatomically grounded. An alternate design that forces the model to learn the right things was proposed by us. Since COVID-pneumonia occurs typically in the peripheral regions of the two lungs, by modifying that region we could even demonstrate the model changing its decision.
Recently, a group in MIT showed that often, the models that predict chest diseases also end up being highly accurate in predicting demographics which is not possible for radiologists. The latter actually signals a deeper problem, namely, model’s bias across race and gender, i.e. the model learns “demographic short cuts” to perform chest diagnosis whose accuracy is uneven across demography.
Can the model explain its decision for a particular image?
Interpretability/explainability of decisions by DL-based AI models is another major concern as it has affected the rate of adoption of AI models in clinical settings. I.e. the number of publications in the area has grown exponentially (to be in several thousands) whereas the number of solutions gaining approvals is a few hundreds and grows at a very slow rate. The Interpretability/explainability is more natural in clinical diagnosis because it is largely multiple evidence-driven. In contrast, most AI models for diagnosis rely only on an image. This scene was largely due to research groups working in silos (ex. vision, natural language processing). However, this is changing.
At IIITH, our group worked on the explainability issue using both multi-modal and only image data. In the first problem, radiology reports (text) associated with an image were leveraged to train a model to diagnose pneumothorax from chest X-rays. Pneumothorax can be very small or large regions of lung collapse. Our design enabled a model to predict pneumothorax by accurately extracting the contour of the pneumothorax region if present; the contour serves as a visual explanation for the decision. In the second problem, a differential diagnosis of malignant melanoma using only images was attempted. Clinicians use multiple information to arrive at a diagnosis. These include a lesion’s appearance with respect to others, location in the body, gender and population-level prevalence. We also designed a clinical-flow-inspired model which performs a lesion-focused analysis and integrates patient and population-level information to finally arrive at a prediction for malignant melanoma. The design specifically allowed interpretability of the final decision by showing how the decision changed with addition of various information.
The Future Scenario
In the future, AI-models need to and are likely to have the capability of mimicking clinical conditions in terms of not only considering multiple sources of information such as lab tests, images, etc., at multiple time points to arrive at robust decisions but also be bias-free and clinically acceptable. Population-specific data is needed to train models to be deployed largely in one geography. Many efforts are on in India, to collect brain data for the Indian population. At IIITH, our interest in brain imaging led us to collect brain scans (MRI) of healthy adults between the age of 20 to 80. This was used to model the structural changes in the brain that occur due to the ageing process. Such normative information is helpful among various things, in the prediction of any cognitive decline, Alzheimer’s disease, etc. at an early stage.
In short, AI in healthcare is here to stay. It’s current role as an assistant to the expert is expected to sustain for long. However, it is likely that in the near future, the role will extend to be a partial /full replacement in non-critical areas.
This article was initially published in the July edition of TechForward Dispatch
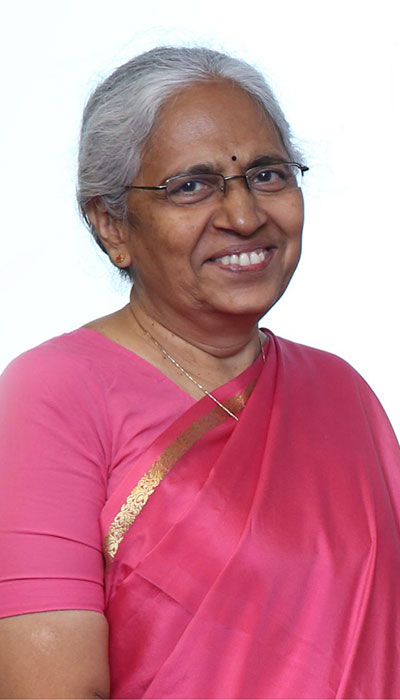
Prof. Jayanthi Sivaswamy is Raj Reddy Professor at IIITH. Trained as Electrical Engineer, she has been working in the medical image computing field for over 15 years. She has extensive experience in developing computer aided diagnostic (CAD) solutions to help screen for eye diseases, lung cancer and Covid based on images from different modalities Besides CAD, her current work focusses on understanding population-based differences in aging of the human brain and developing VR-based solution for anatomy education.
Next post