Continuous monitoring of residents and infrastructure is a given especially with the global push on smart cities. Researchers at the International Institute of Information Technology Hyderabad present solutions to obvious concerns of how humongous amounts of CCTV camera footage can be efficiently stored and analysed.
According to the latest surveillance camera statistics, outside of China, Indian cities of Hyderabad, Indore and Delhi feature among the top 10 most surveilled cities based on cameras per 1,000 people. And this data is based only on public CCTV cameras, installed by the government, ignoring the private network of which there is a sizeable number too. A huge challenge in deploying such large scale camera networks concerns storing videos streamed by them and extracting relevant information in real time. In a study titled, ‘A Cloud-Fog Architecture for Video Analytics on Large Scale Camera Networks Using Semantic Scene Analysis’ that was presented at CCGrid 2023 – the 23rd IEEE/ACM International Symposium on Cluster, Cloud and Internet Computing, IIITH researchers have proposed a scalable video analytics framework that checks all the problem boxes.
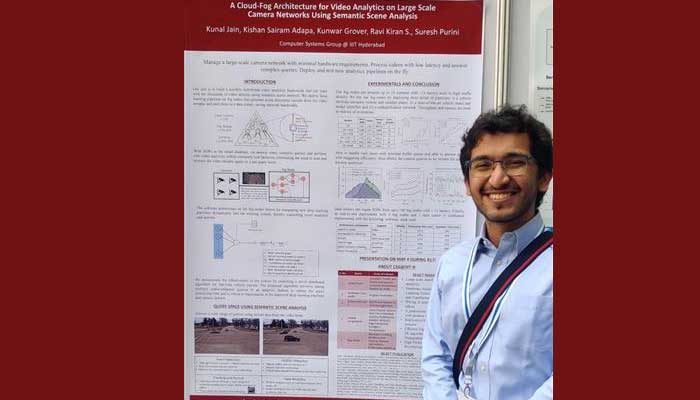
According to Kunal Jain, 5th year Dual Degree student in Computer Science and the primary author of the research paper, although large scale CCTV camera networks are found everywhere, from the streets to malls and industrial spaces like factories and so on, the team focused only on publicly available CCTV cameras installed on road networks. Their aim was to engineer an easy-to-deploy, scalable and loosely coupled hardware-software distributed infrastructure that could process information related to events that don’t require real-time processing such as jumping of red lights, as well as those requiring real-time processing of data such as detection of traffic jams due to malfunctioning traffic lights or accidents and so on. In addition to these, the researchers efforts focused on one of the most complex queries in video analytics which is tracking and pursuit of vehicles that could be a combination of both a real-time pursuit or an offline one, along with data analytics such as rush hour analysis, correlation between traffic and air quality and so on.
What They Did
“An average CCTV camera that has a 1080 pixel resolution generates 72 MB of data per hour; 1,000 such cameras generate 72GB data per hour which is about 2 TB in a day. With 50,000 cameras, we can see that data generated is about 100 TB in a day which is ginormous!”, exclaims Kunal explaining that one of their motivations was to come up with a solution to save on network bandwidth. To achieve that, the work focuses on two things primarily – semantic scene analysis and cloud-fog architecture. The former refers to the way machines understand visual scenes – through object recognition – and establish relationships between objects by generating a textual description of the same. This is especially useful when retrieving images because the textual query search throws up the appropriately captioned image. “In our system, all visual information is stored in textual format known as Scene Description Records (SDR). For instance, in the road setting, if there’s an image of two black cars at an intersection with one of them taking a right turn because of a free right, a query for “a black car at an intersection” will retrieve this image,” says Kunal. Transmitting a textual description instead of an image itself saves network bandwidth and avoids congestion at the ingestion points of the cloud.
In order to create a distributed infrastructure architecture, what they next did was to separate the processing layer and the storage layer in the SDRs. “A fog node is a very small processing unit that quickly processes information given and forwards it to the nearest data centre,” elaborates Kunal. By connecting a few tens of CCTV cameras to one particular fog node, and many fog nodes in the same geographical vicinity to a mini data center, and many mini data centers in turn connected with each other, the researchers created a multi-data center with fog node architecture.
Low Compute, Flexible and Accurate
For the purpose of testing out the system, four different deep learning pipelines were deployed on the fog nodes – object recognition, vehicle tag extraction, vehicle colour, make and model detection and more. “What’s novel in our approach is that if there’s a new ask, for say, identifying jaywalkers on the road, you don’t have to restart the entire system and begin afresh. Individual computational components are broken down so that any new incoming pipeline can subscribe to the ones in common and also add any new tasks on the fly to the system,” remarks Kunal. The team demonstrated the effectiveness of their system in a query on real-time vehicle pursuit with queries getting answered in less than 15 milliseconds. “Our best algorithm was able to track the car between 80-100% of the time,” says Kunal. While the research has focused on road camera networks, the principles can just as well be applied to other large scale surveillance networks too.
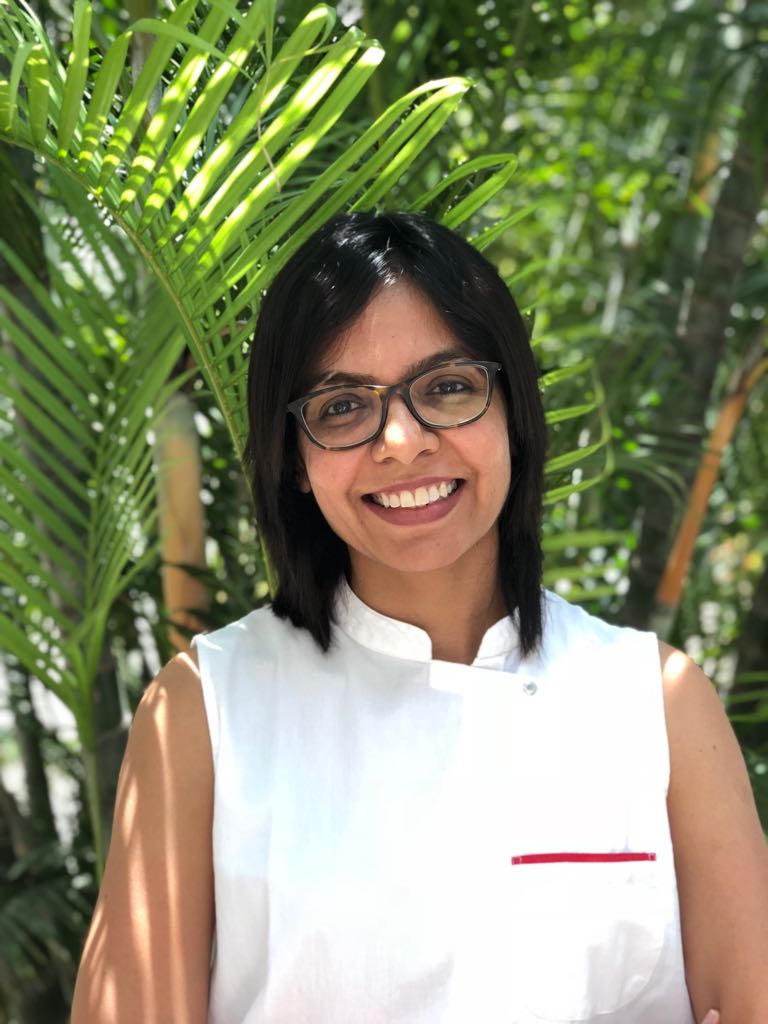
Next post