The International Institute of Information Technology Hyderabad is known for its research driven culture with a focus on AI and ML. But while you think AI and ML is all about computer vision, robotics, and IoT, here’s a sneak peek into the institute’s AI-powered data-driven drug discovery unfolding at iHub-Data centre.
A couple of decades ago, when computers were put to the task of drug design, it heralded a new era in the drug discovery process. Automating the process of screening a large number of molecules to find those eliciting the required biologic response reduced the cost, effort and the time of the drug discovery pipeline. But now with AI in the picture, its increasing use in the pharma industry is driving innovations, improving productivity, and accelerating manifold the process of drug discovery. At the International Institute of Information Technology Hyderabad, one of the key aims of the healthcare vertical at iHub-Data is to accelerate the process of drug discovery through data-driven technology. iHub-Data (https://ihub-data.iiit.ac.in) is a Technology Innovation Hub that has been established under the National Mission on Interdisciplinary Cyber Physical Systems (NM-ICPS) in the area of data driven technologies. The Hub aspires to coordinate, integrate, and amplify basic and applied research in broad Data-Driven Technologies as well as its dissemination and translation across the country.
Efforts in the direction of drug discovery were formally launched in October 2020 with the objective of conducting research in the field of modern Machine Learning methods applied to Computational Chemistry and Drug Discovery. It boasts of an interdisciplinary team of faculty, and researchers drawn from the Centre for Computational Natural Sciences and BioInformatics, the Machine Learning Lab and the Centre for Visual Information Technology.
Faster And Cost-Effective
Speaking of how AI supports the drug discovery process, Prof. Deva Priyakumar, the Academic Head of IHub-Data, says that on an average the lifecycle of a drug takes about 12 to 15 years. “It takes that long because of the kind of expertise that is needed during every phase of the drug discovery pipeline. From biologists and virologists to genomists, there are many different kinds of specialists involved in the process in a serial fashion for the pipeline to be successful,” he says. The earliest stage of drug discovery deals with the identification of potential molecules from the large existing pool of molecules that can function as targets for certain diseases. The later stages revolve around developing these molecules into viable drugs by first conducting preclinical trials, before moving on to clinical trials. In the past, innovations in the drug discovery pipeline happened in a piece-meal fashion. Where physics-based methods were initially used to design molecules, traditional computational methods began to be applied and this happened somewhere in the middle of the pipeline. However according to Prof. Priyakumar, “In the last 3-4 years, instead of a fragmented approach by different experts, we have AI coming in that supports every stage of the drug discovery process making it entirely seamless and more efficient. At the iHub-Data centre, we have developed close to more than 10 different ML methods that have direct relevance in the drug discovery pipeline”.
Exploration of Chemical Space
Traditional methods have limitations in the number of drug molecules that can be analysed as potential hits for a particular disease. AI and Machine Learning in particular helps break these limits by enhancing exploration by a factor of billion and higher, increasing the chances of finding a better drug. Dr. Girish Varma who is leading the ML efforts behind the drug discovery process at iHub-Data observes that unlike algorithms that are used for image, speech or text processing, the ML techniques in Sciences involve a number of intricacies. “Since they deal with molecules and compounds, the modelling requires novel approaches too.” The work carried out by a team of researchers under the guidance of Prof. Priyakumar has led to the successful filing of a patent for the ‘System and Method for Exploring Chemical Space During Molecular Design Using a Machine Learning Model’.
Understanding Drug Properties
Before a drug can be approved for human usage, it goes through preclinical and clinical trials. While clinical trials refer to experiments performed on humans, the preclinical stage is when wide doses of the drugs are tested for efficacy and safety either through cell cultures or on animals. “Traditionally these methods are very expensive and conducted in labs. AI can help drastically bring down the number of experiments needed to be carried out, reducing the time and cost involved,” says Siddhartha Laghuvarapu, Research Engineer at iHub-Data.
Understanding The Biology
The classic way of representing molecules is via chemical formulae. “A chemical formula can’t tell if a particular molecule can block or attach itself to another. But with the help of the chemical notation, we use ML techniques to create 3D representations of the compounds. 3D depictions are useful in visualising interactions between proteins,” says Dr. Varma, adding that they also help in identifying which part of the protein the drug may bind itself to. Simplistically put, before a drug can be designed to treat a disease condition, it is essential to understand the mechanisms by which the drug can interact with the relevant proteins. The algorithms help in identifying these binding sites that potential drugs can interact with.”
Developing Datasets
For any machine learning to take place, datasets are an integral part of the exercise. One of the major efforts undertaken by iHub-Data is the development of a large-scale dataset that can be used for creating machine learning models to evaluate whether a given molecule has the potential to be a drug or not. Explaining how the design of a new drug molecule depends on the way in which it interacts with certain proteins in the human system, Prof. Priyakumar says, “This dataset will enable researchers to quantify how strongly a molecule will bind to a protein.”
The Drug Discovery team at iHub-Data is also working on creating a dataset specifically for protein kinases. The latter are known to regulate all aspects of cell life, and any aberrations in their genes causes cancer, as well as early ageing among other issues in humans. With increasing drug resistance to kinase inhibitors, kinase drug discovery is a very active area of pharmacological research. “We started a project to develop binding affinity datasets of kinase-ligand complexes recently in collaboration with a company, InSilico Medicine, a Hong-Kong-based biotechnology startup,” says Prof. Priyakumar.
Identifying Drug Interactions
There are certain drug combinations that do not react favourably and ought to be avoided by patients. “When the action of one drug affects the other either positively or negatively, it’s known as a drug interaction,” says Prof. Priyakumar citing the example of Aspirin and Warfarin. “Aspirin is a common drug originally used as a non-steroidal anti-inflammatory agent, but in the last 30-40 years, has been prescribed as a blood thinner. Warfarin is a blood thinner too. If someone takes Warfarin for a cardio vascular condition and the same patient takes an Aspirin for a headache, the blood thins too much and it may cause internal bleeding and other complications.” As part of the drug discovery process, the ML methods developed by the team also assist in predicting if the new molecule being identified will interact with any of the existing drugs or not.
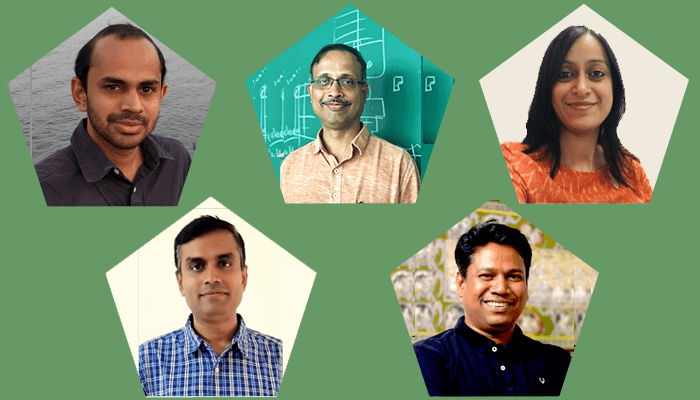
In The Pipeline
With the data driven drug discovery platform providing ample opportunities for research and learning, the team’s efforts have borne fruit with publications in leading journals and conferences. In addition to this, there’s an ongoing academic collaboration with other institutes and experts from industry in the form of a seminar series. For the group though, the larger goal is that of translating all the research efforts into real-life solutions. “Discussions are on with multiple partners on kicking off therapeutic programs jointly. This will involve identifying a disease and then coming up with an appropriate molecule to treat it,” says Prof. Priyakumar.
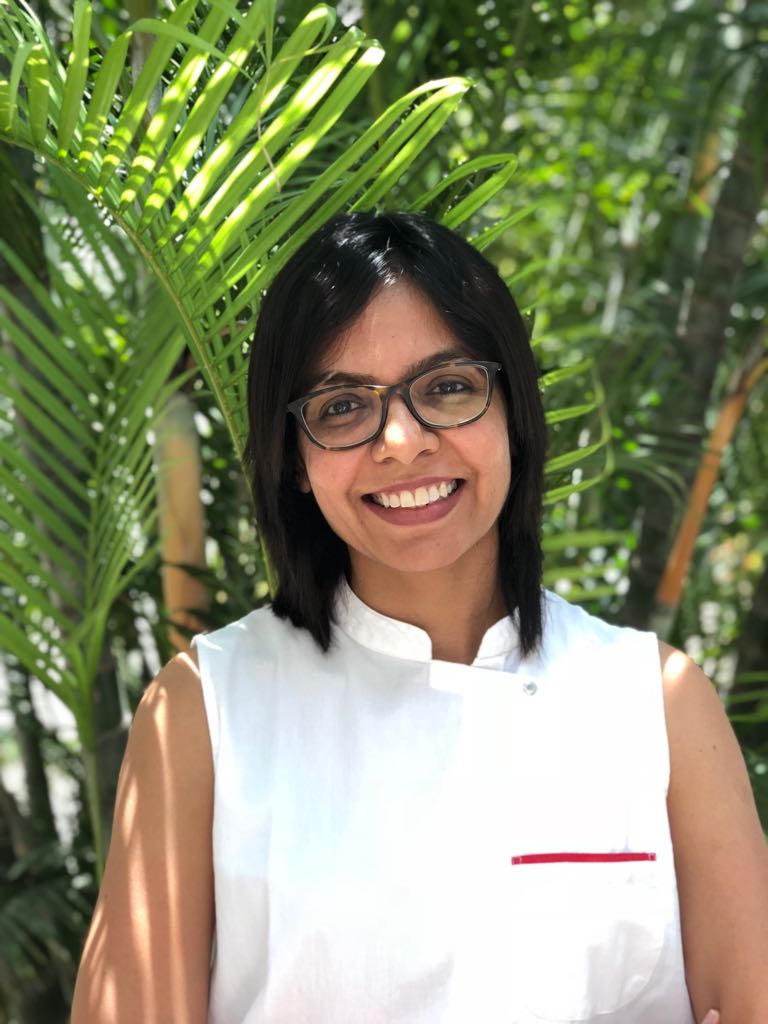
Next post