The solution which combines robotics and computer vision can automate the parameter data collection process that currently relies on manual assessment of structures for earthquake vulnerability.
Automation in almost every industrial domain has demonstrated its superiority by virtue of being faster, cheaper and in most cases, more accurate too. Researchers from IIITH reveal its application in the building inspection domain.
There’s an oft-repeated saying among earthquake engineers: Earthquakes don’t kill people; buildings do. Hence following the preventive care approach, the National Disaster Management Authority relies on manual surveys that double up as preliminary diagnostic tools of existing buildings to determine their ‘health’. “There’s a standardised checklist known as the Rapid Visual Survey (RVS) document that is used by surveyors to assess potential risk of structures,” explains Prof. Pradeep Ramancharla, former Head of the Earthquake Engineering Research Centre at IIITH. However sampling even 2-3% of the 5-10 lakh buildings in cities is an uphill task and an aerial-based survey for visual inspection of buildings was initially mooted. It has since led to an interdisciplinary series of drone experiments by researchers from the Robotics Research Centre (RRC) and the Centre for Visual Information Technology (CVIT) demonstrating accuracy, speed and scalability of this novel solution. It also resulted in the publishing of a paper titled ‘UAV-based Visual Remote Sensing for Automated Building Inspection’ that was presented at the Computer Vision for Civil and Infrastructure Engineering (CVCIE) Workshop conducted on the sidelines of the prestigious European Conference on Computer Vision (ECCV) 2022.
What It Does
Explaining that their proposed solution does not rely on any specific UAV, Prof. Harikumar Kandath of the Robotics Research Centre says, “For this project, we used primarily two sensors that can be mounted on any UAV – an RGB camera – that gives coloured images by capturing light in red, blue and green wavelengths, and a range sensor. The latter is similar to a laser pointer which calculates distance from the pointer to wherever it points.” Parameters such as dimensions of the building plan, height of the buildings, number of storeys each building possesses, distance between buildings and so on are factors essential for seismic-risk assessment of structures. To collect this information, the team’s UAV conducted sorties that captured images of buildings in 3 modes – a frontal, in-between (buildings) and a roof mode. While the frontal and in-between modes uses a forward-facing camera, the roof mode deploys a downward-facing camera to estimate the plan shape of the buildings, the roof area and layout as well as the objects on the roof such as solar panels, water tanks which are potential seismic hazards when present in large quantities. “Images taken by the drone are ‘stitched’ together to prevent overlap of the same image(s) and information such as the number of windows, storeys and so on is obtained,” says Prof. Ravi Kiran S from the Centre for Visual Information Technology (CVIT). He adds that what’s more interesting is the ability of the drone to capture data not available from mere 2D imagery such as the height of the building or height of each window from the ground, size of windows and more.
Testing It Out
Accuracy of the team’s algorithm for distance estimation revealed an error of less than 1%. “We found that for this particular objective of measuring distance between adjacent buildings, our model was performing better than Google Earth,” says Kushagra Srivastava, Research Assistant at RRC. Similarly for plan shape estimation and roof layout estimation, when compared with Google Earth, a difference of 4.7% was obtained. “If a single person tries to calculate what percentage of a roof is occupied with objects, it will take several days whereas 2 minutes of flight data can be processed to get the same information instantaneously,” observes Prof. Harikumar. According to Prof. Ramancharla, currently the solution helps in automatically estimating 1/3rd of the visual assessment parameters as laid out in the RVS document.
Beyond Mere Code
“The real significance of this project is that it brings together the components of Vision and Robotics in a very organic manner. So, in a sense, it is closer to what is expected of the so-called AI system today,” remarks Prof. Ravi Kiran. By virtue of borrowing several components and elements of the project from the Open Source world, the team has in turn released their source code on GitHub at https://uvrsabi.github.io/. “Along with data collection instructions, there are also software installation do’s and don’ts. The system is GUI-based where any user inputs the video, selects the module required, such as the frontal mode or the roof mode and gets the results,” explains Kushagra. The ease of use and simplicity of the software suite was especially designed keeping in mind those not familiar with Robotics. “Our target audience is those from the civil engineering field. Hence our focus was not just on developing software but also in putting together documentation and tutorial videos so that it would be really easy for adoption by anyone,” notes Prof. Ravi Kiran.
One Drone, Many Uses
While diagnostic information about structures may encourage building owners to retrofit buildings to strengthen their safety, such information may be useful for other kinds of civil inspection works too. “We could use this drone technology to visually inspect bridges, especially those in locations that are hard to access, across rivers and in high altitudes. It could also assist in detection of cracks in buildings, presence or absence of fire exits and so on,” says Prof. Ramancherla, musing that in future the drones can even aid in undertaking repair works. “Maybe if there is any paint or chemical injection required to strengthen a building, it can be done through the drone itself.”
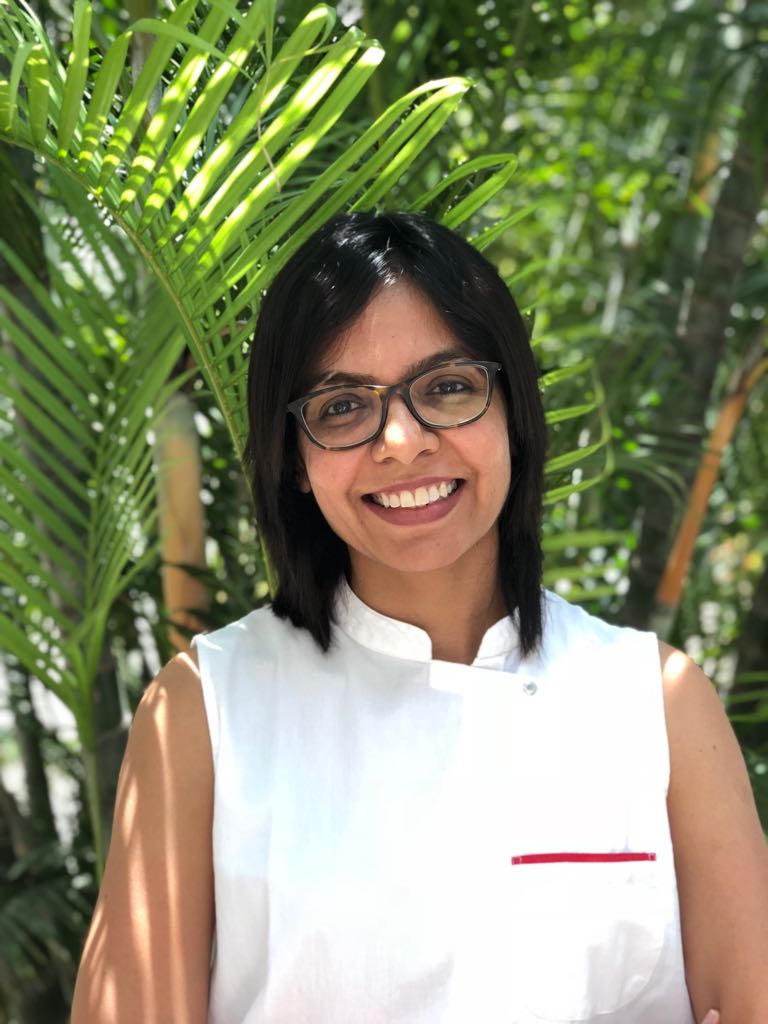
Next post