The study has been accepted for presentation at the 2023 Conference on Computer Vision and Pattern Recognition at Vancouver from June 18-23.
Teaching a machine to recognise and correctly interpret human emotions and mental states has been a pursuit of the evolving field of affective computing. With a new ML model that analyses emotions from complex movie scenes, researchers from CVIT have taken AI closer to the goal.
Understanding Mental States
In a study titled, “How you feelin’? Learning emotions and mental states in movie scenes”, primary author Dhruv Srivastava along with co-authors Aditya Kumar Singh and Prof. Makarand Tapaswi introduce a machine learning model that relies on a Transformer-based architecture to understand and label emotions not only for each movie character in the scene but also for the overall scene itself. “Emotion understanding in the visual domain has been dominated by analysing faces and predicting one of Ekman’s 7 basic emotions. In the last few years, text-based emotion analysis has also developed where dialog is used to understand the mental state of the character,” explains Dhruv. With cinema possessing a vast amount of emotional data mirroring the complexities that exist in everyday life, the research group embarked upon movies as their starting point. Unlike static images though, movies are extremely complex for machines to interpret. “A character can go through a range of emotions in a single scene – from surprise and happiness to anger and even sadness. Therefore the emotions of a character in a scene cannot be summarised with a single label and estimating multiple emotions and mental states is important,” says Dhruv. Prof. Tapaswi makes a distinction between an emotion and a mental state by clarifying that while the former can be explicit and visible (e.g., happy, angry), the latter refers to thoughts or feelings that may be difficult to discern externally (e.g., honest, helpful). Besides, he says, decoding emotion and mental state based only on the language used is fraught with difficulty. Take the statement, “I hate you”. Interpreted in isolation, bereft of visual cues, a machine will likely label the underlying emotion as ‘anger’. However, the same statement could be uttered in a playful manner where the character is smiling at another while saying it thereby confusing machines.
What They Did
To train their model, the team of researchers used an existing dataset of movie clips collected by Prof. Tapaswi in his previous work called MovieGraphs that provides detailed graph-based annotations of social situations depicted in movie scenes. So in effect, EmoTx was trained to accurately label emotions and mental states of characters in each scene through a 3-pronged process – one, by analysing the full video and the actions involved, two, by interpreting individual facial features of various characters and three, by extracting the subtitles that accompanied the dialogues in each scene. “We realised that combining multimodal information is important to predict multiple emotions. Based on the three criteria, we were able to predict the corresponding mental states of the characters which are not explicit in the scenes,” shares Aditya. Upon qualitative analysis, what turned out to be fortuitous for the researchers is their model’s ability to predict when the individual character displays the emotions.
Complex And Real
Teaching a machine to interpret emotions from movies may not be entirely novel but what makes the IIITH research stand out is that unlike previous works that predict a single emotion from a movie scene, the former considers multiple emotion labels. Even in cases that have multiple emotions, the datasets rely on constrained environments where emotions are extracted based on facial features only. “Our movie dataset mimics situations in the real world and consists of real-life scenarios that are extremely complicated,” emphasises Dhruv, adding that a similar ML model based on the F.R.I.E.N.D.S TV series exists. The EmoTx model is however trained on a large number of movies of different genres and scenarios. Prof. Tapaswi discloses that while there is much work to be done yet, the model achieves decent performance for frequently occurring movie emotions such as excited, friendly, and serious. “However, estimating mental states such as calm, honest, and helpful remains challenging,” he admits.
Use Cases
With the exponential rise in the availability of audiovisual content and its consequent consumption, it’s imperative to have accurate content summarization and recommendations that can help viewers in making the right choice. “With a better understanding of the underlying emotions, a better tagging system can be applied to online content which in turn may lead to better recommendation systems,” remarks Dhruv. According to the team, the model can also be leveraged to assist in the field of personal healthcare. “If we can teach machines to accurately identify human emotions, they can understand and interact better with humans, perhaps as healthcare agents in assisting people with managing their own moods and so on,” says Dhruv. While the model has been based on vision and language, the team envisages a future iteration that could include an audio component. The tone and timbre of how something is said is known to provide a better reference to the emotional state of the concerned speakers.
To know more about the study, click here and here.
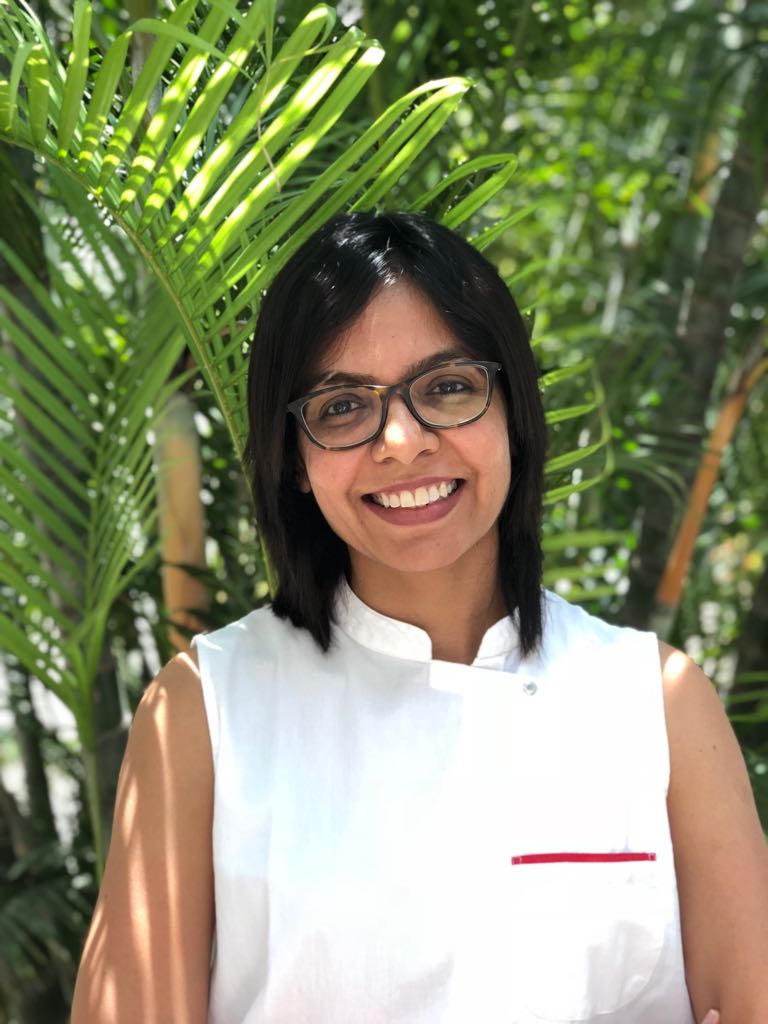
Next post