Researchers from IIITH analyse Ahmedabad traffic e-challans data and discover interesting patterns in motorists’ behaviour both in terms of repeat offenses as well as fine payment.
– Traffic offenders with more number of e-challans are less likely to pay fines
– Payment of smaller fines preferred over larger ones
– ML model trained on dataset predicts which vehicle is likely to commit a traffic offense in the near future
Last semester at IIITH, a novel elective course titled ‘Big Data and Policing’ drew over 60 odd students. Taught by Prof. Ponnurangam Kumaraguru (PK), an Associate Prof at Indraprastha Institute of Information Technology, Delhi and current Adjunct Faculty at IIITH (who was a full-time faculty at IIITH on sabbatical when the course was offered), it saw many interesting projects that culminated in an interactive poster presentation session. But one of the classroom projects undertaken by students Aanshul Sadaria, Kanay Gupta, Shashank Srikanth, Hiimanshu Bhatia, and Pratik Jain under Prof. PK’s guidance, went on to bear fruit in a research paper titled ‘Don’t Cross That Stop Line: Characterizing Traffic Violations In Metropolitan Cities’ that has now been submitted for review in a conference. For Prof. PK whose expertise lies in privacy and security in online social media, or “anything in society at large, where social computing can come in handy,” he confesses that he gets excited about Data Science for social good. Or in this specific case, traffic data whose analysis can help rein in the number of road accidents due to violations.
Electronic Challans
With the Digital India initiative picking up steam and e-governance more of a reality than before, the Indian Police Department too is also making use of Information and Communication Technology (ICT) in monitoring the law and order situation. Big data is being used to note down trends and patterns in the behaviour of crimes with the intention of effectively reducing and preventing such crimes. But apart from serious crimes like murders and robberies, traffic violations too have come under the big data radar. In fact, one of the ways in making traffic police more efficient is in the manner in which traffic violations are recorded and dealt with. Thanks to the CCTV cameras installed at various traffic signals across many Indian states and cities, the Indian police is able to track traffic offenses via a non-contact method where traffic footage identifies the offending vehicle based on the license plate. And in lieu of a paper ‘ticket’ or receipt issued according to the type of traffic offense committed under the Motor Vehicles Act, 2019, offenders now receive electronic challans or e-challans where they are liable to pay an appropriate fee either online or offline.
Big Data and Policing
For the authors of the research paper, the goal of their project was to see how such digitized data on traffic violations could be analysed to provide useful feedback to improve the system. “With more and more cities moving to e-governance, we wanted to see how to provide useful insights to assist in governance and policing,” says Aanshul Sadaria, one of the researchers.
An Ahmedabadi, Aanshul himself had been at the receiving end of traffic challans having recorded a few transgressions that he explained after some self-analysis. “There was a pattern to how the e-challans had been recorded for me. They were at 3pm and at a particular location, because that was when I used to go to my classes, through a particular route. Typically being late to class, I was prone to committing the offense of jumping a red light. That’s how I thought we could use data available on the Ahmedabad e-challan website and find similar interesting patterns for the general public,” he says.
Gathering Data
“Not only did the Ahmedabad police have the most robust kind of a system, but it was also easier to access data online here versus other metropolitan cities where data was secured through captcha,” explains Kanay Gupta, another student involved in the research. The researchers collected data on all kinds of traffic challans generated from September 2015 through August 2019. “ While Gujarat has a prefix of GJ and Ahmedabad has city number registered as 01, we brute forced through all possible combinations of vehicle registration numbers from AA00 to ZZ99 to find information about challans generated, if any, against every single vehicle registered there,” says Aanshul. In this manner, a dataset of over 3 million e-challans was generated. Every e-challan documented has information about location, time, date, type of traffic violation, amount of fine levied and whether it has been paid or not.
Traffic Transgression Trends
From the total number of e-challans found in the dataset, the research team segregated the number of paid versus unpaid challans. A whopping 59,24,30,400 cr is still outstanding to the government as on August 22, 2019. Challans were also characterised based on the type of offense committed – red light violation, riding without a helmet, improper parking, stop line violation, driving without a seatbelt and so on. It was found that jumping a red light accounted for the most number of challans recorded. By analyzing the date and time of challan issual, the researchers discovered a pattern of higher or lower incidences of violations corresponding to celebrations in the city such as festivals or other similar events. When e-challans issued were zero during events such as Rath Yatra, it meant that the police personnel were on security duty. However, during other festivals such as Ganesh Chaturthi and Navratri, there was a notable rise in the number of challans issued. A spatial analysis of the challans also revealed certain hotspots in the city where violations were more likely to occur.
Predicting Repeat Offenses
Apart from plotting trends, the team trained a machine learning model based on the history of registered vehicles getting challans. “Similar to how big data is being used in policing of big crimes, we have made an attempt to predict how likely a traffic offender is to commit an offense again. We achieved 95% accuracy in predicting violations,” says Kanay. The researchers could narrow down on features that could predict a vehicle owner’s tendency to repeat an offense – known as ‘recidivism’. The features included unpaid number of challans: the higher the number of such challans, the greater the chances of repeat offenses, recency of the violation or number of days since last challan was issued and so on.
Implications
Insights from this study were handed over to the Ahmedabad police, who requested a similar analysis for the city of Rajkot. “With these kind of findings, the Police can get a sense of the violations that are taking place and the locations where they are happening. It will help in planning where they should dispatch their set of officers. In fact, I foresee that this kind of data can even be used for city planning. The answer to the question of how do I design my roads can actually come from this,” says Prof. PK. With the new Motor Vehicles Act (Amendment) 2019, that came into effect from September 1, 2019 and its focus on enhancement of penalties for driving errors as well as violating other road regulations, such an analysis would be crucial. Similarly under the recent road-rationing scheme introduced by the New Delhi government where vehicles with odd-numbered license plates are allowed to ply on the roads on certain days and even-numbered license plates on others, patterning trends in traffic e-challans would be beneficial. “It would be nice to see the data before the implementation and after the implementation, just to show the effectiveness of the rules” says Prof. PK.
For more details on the research paper, see: Don’t Cross That Stop Line: Characterizing Traffic Violations In Metropolitan Cities
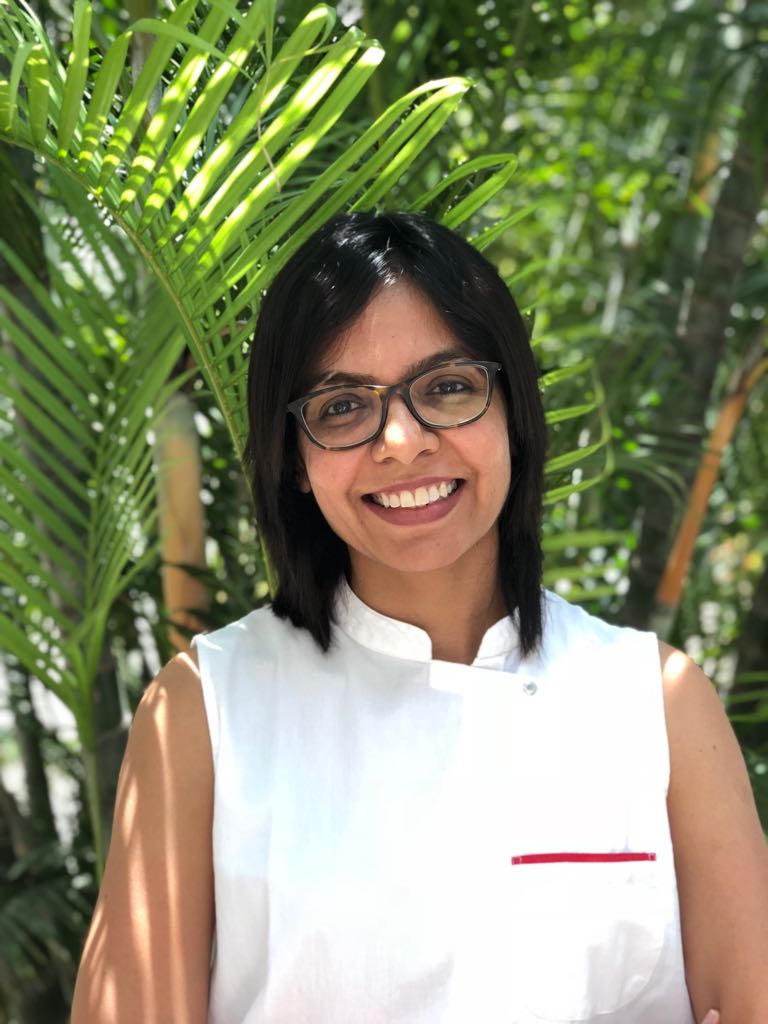
Next post